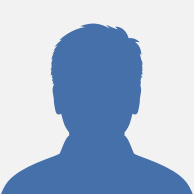
Critical lifeline infrastructure network hubs are examples of soft targets and crowded places that, if impacted, can result in severe direct and indirect losses to the public, society, and the economy. For example, multi-modal interdependent transportation networks characterized as cyber-physical infrastructure systems, while contributing to efficient mobility and connectivity in urban regions, may also lead to adversarial opportunities for compromise. Developing data-driven methods for quantifying the risk and resilience of such networked cyber-physical systems is a challenging research problem. This project will address this challenge by uniting innovations in interpretable machine learning with behavioral simulation models to quantitatively enhance threat deterrence and risk mitigation at transportation network hubs with an initial proof-of-concept application to urban rail networks. Rail networks – including and especially subway systems – are critical lifecycles for urban, regional, and national transportation and supply chains, but rail stations are soft targets for physical, cyber, and cyber-physical attacks. As indicated in a report by the Presidential Committee of the National Infrastructure Advisory Council, intentional man-made attacks may attempt to opportunistically leverage natural hazards such as floods when service levels are down. The owners and operators of railway systems need to assess the extent and severity of damage caused by disruptions to the service provided by the entire rail network when one or more stations are targeted and how effectively and quickly the system can fully bounce back. This assessment may be critical for the allocation of resources, such as law enforcement personnel and surveillance sensors, to each soft target or station. From the perspective of the attacker, targeting certain stations can cause more direct damage as well as cascading failures along the rail networks leading to indirect damage. The more the net damage, the more the desirability as a target. Thus, the threat increases with the attacker’s perception of the damage that a hit on a specific station can cause. The total risk is a function of the threat, vulnerability, and consequence, as well as the recovery potential, while the direct and indirect consequence itself has feedback on the threat levels and perceptions.
Current goals are to fully assemble the project team, further set up the problem space, gather initial data, and take preliminary steps towards developing solution frameworks and data-driven methods in machine learning, network science, and agent-based systems for threat deterrence in the soft targets of rail stations. We are not aware of any research that attempts to develop quantitative methods to characterize the threat as a function of direct and indirect damage, especially in the context of rail networks, and develop a deterrence strategy. The attacks on the London metro and other rail networks around the world show that the threat is real and that the Homeland Security Enterprise must be prepared. Rail transport is listed as a national critical function set by the Cybersecurity and Infrastructure Security Agency of the Department of Homeland Security. Our approaches and insights can inform threat deterrence assessments and dynamic, regional resource allocation strategies for mitigating threats.